
cutting down iteration time from ~250ms / iter to 135ms / iter.
#128 TUNING FORK MEDICAL CODE#
The improvement from the one line of code is noticeable, e.g. At the time of writing (Dec 29, 2022) this makes pile() available in the nightly release. Note that the code by default uses PyTorch 2.0. It's identical to what happens in the meat of the training loop of train.py, but omits much of the other complexities. efficiency notesįor simple model benchmarking and profiling, bench.py might be useful. $ python sample.py -start=FILE:prompt.txt. You can also prompt the model with some text from a file, e.g. If you'd like to sample from a model you trained, use the -out_dir to point the code appropriately. start="What is the answer to life, the universe, and everything?" \ Finetuning can take very little time, e.g. Unlike OpenWebText this will run in seconds.
#128 TUNING FORK MEDICAL HOW TO#
For an example of how to finetune a GPT on new text go to data/shakespeare and run prepare.py to download the tiny shakespeare dataset and render it into a train.bin and val.bin, using the OpenAI BPE tokenizer from GPT-2.

finetuningįinetuning is no different than training, we just make sure to initialize from a pretrained model and train with a smaller learning rate. This then becomes the more appropriate baseline w.r.t. Indeed, taking the GPT-2 (124M) checkpoint and finetuning on OWT directly for a while reaches loss down to ~2.85. This means there is a dataset domain gap. However, we have to note that GPT-2 was trained on (closed, never released) WebText, while OpenWebText is just a best-effort open reproduction of this dataset. We can get the numbers as follows:Īnd observe the following losses on train and val: model OpenAI GPT-2 checkpoints allow us to get some baselines in place for openwebtext. You'll most likely want to tune a number of those variables depending on your needs. Have a look at all of its args, the script tries to be very readable, hackable and transparent. We can sample from the model by simply $ python sample.py.įinally, to train on a single GPU simply run the $ python train.py script. By default checkpoints are periodically written to the -out_dir. Your multinode training will work, but most likely crawl. In particular, if you don't have Infiniband then also prepend NCCL_IB_DISABLE=1 to the above launches. It is a good idea to benchmark your interconnect (e.g. Run on the first (master) node with example IP 123.456.123.456: This still runs in about ~3 minutes, but gets us a loss of only 1.88 and therefore also worse samples, but it's still good fun: Because our network is so small we also ease down on regularization ( -dropout=0.0). We'll also use a much smaller Transformer (4 layers, 4 heads, 128 embedding size), and decrease the number of iterations to 2000 (and correspondingly usually decay the learning rate to around max_iters with -lr_decay_iters). Then when we evaluate we get a bit more noisy but faster estimate ( -eval_iters=20, down from 200), our context size is only 64 characters instead of 256, and the batch size only 12 examples per iteration, not 64. Here, since we are running on CPU instead of GPU we must set both -device=cpu and also turn off PyTorch 2.0 compile with -compile=False. $ python train.py config/train_shakespeare_char.py -device=cpu -compile=False -eval_iters=20 -log_interval=1 -block_size=64 -batch_size=12 -n_layer=4 -n_head=4 -n_embd=128 -max_iters=2000 -lr_decay_iters=2000 -dropout=0.0 But even without it, a simple train run could look as follows: I recommend getting the bleeding edge PyTorch nightly ( select it here when installing) as it is currently quite likely to make your code more efficient. No worries, we can still train a GPT but we want to dial things down a notch. I only have a macbook (or other cheap computer). Better results are quite likely obtainable by instead finetuning a pretrained GPT-2 model on this dataset (see finetuning section later). Not bad for a character-level model after 3 minutes of training on a GPU.
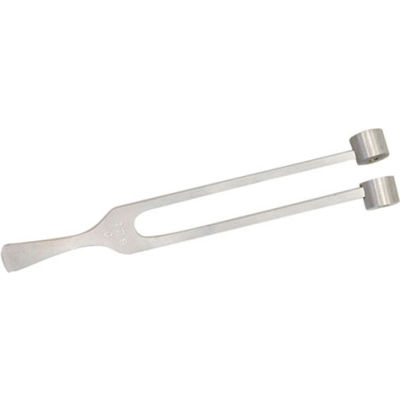
To end his power, the day of thrust for a common men If you have done evils of all disposition And what have you tyrannous shall do this?
